Decoding the use of ChatGPT – and similar LLM applications – after the dust settles: Applications of AI in Pharma and the Life Sciences industry

AI/LLM in the form of ChatGPT, Perplexity (referred to as AI in the text later) and similar have resulted in a transformational impact in the way information is researched and used.
We can now instantaneously be presented with a summary of our query and are allowed to ask follow-up questions using natural language, build on our understanding sequentially and access reference checks if required. These AI tools have strengths and limitations like any other tool and can be very potent when used by people with right knowledge and expertise.
AI, like all technologies in the past is met with two types of reactions. Excessive optimism of assuming it to be the solution for all problems/challenges in work or pessimism and paranoia of AI being wrong in results, breaching all regulations etc. Instead it requires a more balanced approach of understanding, assessing and ensuring its use for the right objectives. All responsible business leaders and technology leaders need to follow this approach.
AI in the Life Sciences industry
The Life Sciences industry involving Pharmaceutical and Biotech companies, consulting partners and vendors working in drug lifecycle from discovery, approvals and eventual launch and access to patients, have been watching the development closely and are keen to ride this latest wave of technology. All stakeholders essentially understand the big picture of ensuring patient safety and bringing efficacious and safe drugs to market. The accuracy of information, especially clinical data, clinical trial data and analysis of data and information is required to be one hundred percent accurate. Small deviations and errors can lead to large errors in final estimations which can eventually lead to wrong interventions being approved or right interventions being rejected.
A number of challenges in current AI offerings have been flagged such as inaccurate data/information, potential IP infringement, biased data, uncertainty around data protection, lack of governance over information being fed/taken out of AI platforms etc. Many Life Science leaders are rightfully sceptical about using AI in their work, but the trick lies in understanding the use case for AI. This does not imply that AI does not have use in high end industries – such as Life Sciences – but careful evaluation is required for appropriate use cases.

Use case for ChatGPT and similar AI tools:
A good method to understand the use case of AI tools in life sciences industry is by drawing a two by two Risk – Impact Matrix.
-
High Impact, High Risk:
-
Low Impact, High Risk:
-
High Impact, Low Risk:
-
Low impact, Low Risk:
The 2 by 2 matrix explains use cases for applications of ChatGPT and similar AI tools in its current form

High Impact, High Risk:
High risk activities for Pharma/Biotech and consulting firms may include using AI tools to create final outputs such as design of clinical trials, creating news alerts for decision making, summarising and analysing clinical trials to estimate efficacy and safety, creating final landscapes and reports etc. Using AI tools such as ChatGPT etc. can significantly increase the speed and efficiency of work but may compromise quality which might lead to additional work compromising all initial gains of efficiency or in worst case scenario may result in providing wrong outputs leading to wrong decision making. Companies would have to be very careful in implementing AI for these high impact activities and would need to implement right governance policies and checks and balances to ensure appropriate use. It is not advisable to implement AI tools in these activities without proper pilot testing etc.
Low Impact, High Risk:
These activities could include trying to finish some aspects of client deliverables quickly using AI without quality check, creating policies/processes using AI etc. These activities can be accomplished using better planning and project management but incorporation of AI without checks and balances can impact the quality of final output which might result in wrong deliverables/outputs and loss of clients and reputation. It is not advisable to implement AI tools without proper policies, processes and governance.
High Impact, Low Risk:
High Impact, Low Rosk activities may include conducting quick research for knowledge building by experts, creating/calculating rough estimates of information, creating disease backgrounds etc., creating draft technical information base for business development, initial client lists, enterprise knowledge management, use by administrative, HR and other support functions for research and work etc. These activities while essential, do not require data and information accuracy to the level of high-risk activities but consume a lot of time. This is where the true transformational potential of AI tools currently exists. AI can improve the baseline knowledge of scientists, consultants, and leaders by providing very quick initial results while the rest of the time is used in developing further deep knowledge and strategy. This ensures highly impactful output in decision making by increasing the efficiency and enabling more time for creative and deep thinking and analysis. It is highly recommended that all firms evaluate immediate implementation of AI in low-risk high reward activities while still ensuring the safety of data and evaluating/addressing appropriate concerns.
Low Impact, Low Risk:
These activities include basic marketing material, basic/introductory training content, internal meeting minutes, preparing internal low impact processes, staff engagement activities etc. These activities do not have the same risk of impact if something is wrong/inaccurate and can enhance the productivity and efficiency of work. It is recommended to implement low-cost AI solutions after assessing return on investment.
General AI tools vs Specific AI tools (ChatGPT vs life science specific tools):
The general AI tools such as ChatGPT etc. are designed to cater to a broader audience and cast a wide net to generate approximate information. Many companies are developing applications using LLMs & other models which are specific, go deep and provide accurate information. It is advisable to focus on both type of tools and applications depending upon the need. There is a huge unmet need for specific tools/applications focused to answer/solve key issues appropriately.
Recent developments in marketed and under development Specific/Targeted AI tools:
Some targeted applications/databases are already available in market that deploy AI in providing quick search of data and they are further building natural language search and summarising capabilities within their existing applications. There is also a push to integrate external sources research with internal knowledge of the organisation and providing an overall summary. The key challenge here remains protection of organisation data, user data and governance.
Several applications are being developed using LLM and other models which can provide specific and accurate results and summaries for targeted areas such as TA landscapes, research of data and summary, data visualisation and infographics, organisational knowledge search using natural language search, targeted clinical landscapes etc. It is important to ensure that risks related to data security, IP infringement, data governance, user management etc.
Governance of AI and its implementation:

Governance of tools and data protection is one of the key challenges that industry leaders face while exploring and using AI tools & platforms. It is important that any AI tool/application using or processing confidential data (in part or full) is governed appropriately by Tech and IT teams of the organisations to monitor the use and ensure compliance with various policies such as GDPR. Managing applications within organisation’s internal IT infrastructure and ensuring appropriate firewalls is the key step. All relevant policies regarding usage and data processing and storage should be drafted by the Tech and IT team in close consultation with legal and scientific teams. In case of operations within an external IT environment, evaluating and agreeing to privacy policies and usage and storage of data is paramount. Companies should evaluate the data processing and storage by applications technically and legally and in case of doubt should only use these applications for “high impact, low risk” and “low impact, low risk activities”.
Conclusion:
Recent advancements in AI will not replace skilled people but instead will allow people to do more impactful work in a shorter amount of time and will further unlock the potential for creativity and innovations. All companies and individual professionals should work towards integrating the AI tools and upskilling themselves to allow for next wave of innovation and transformation.
Author – Kunal Srivastava
August 2024
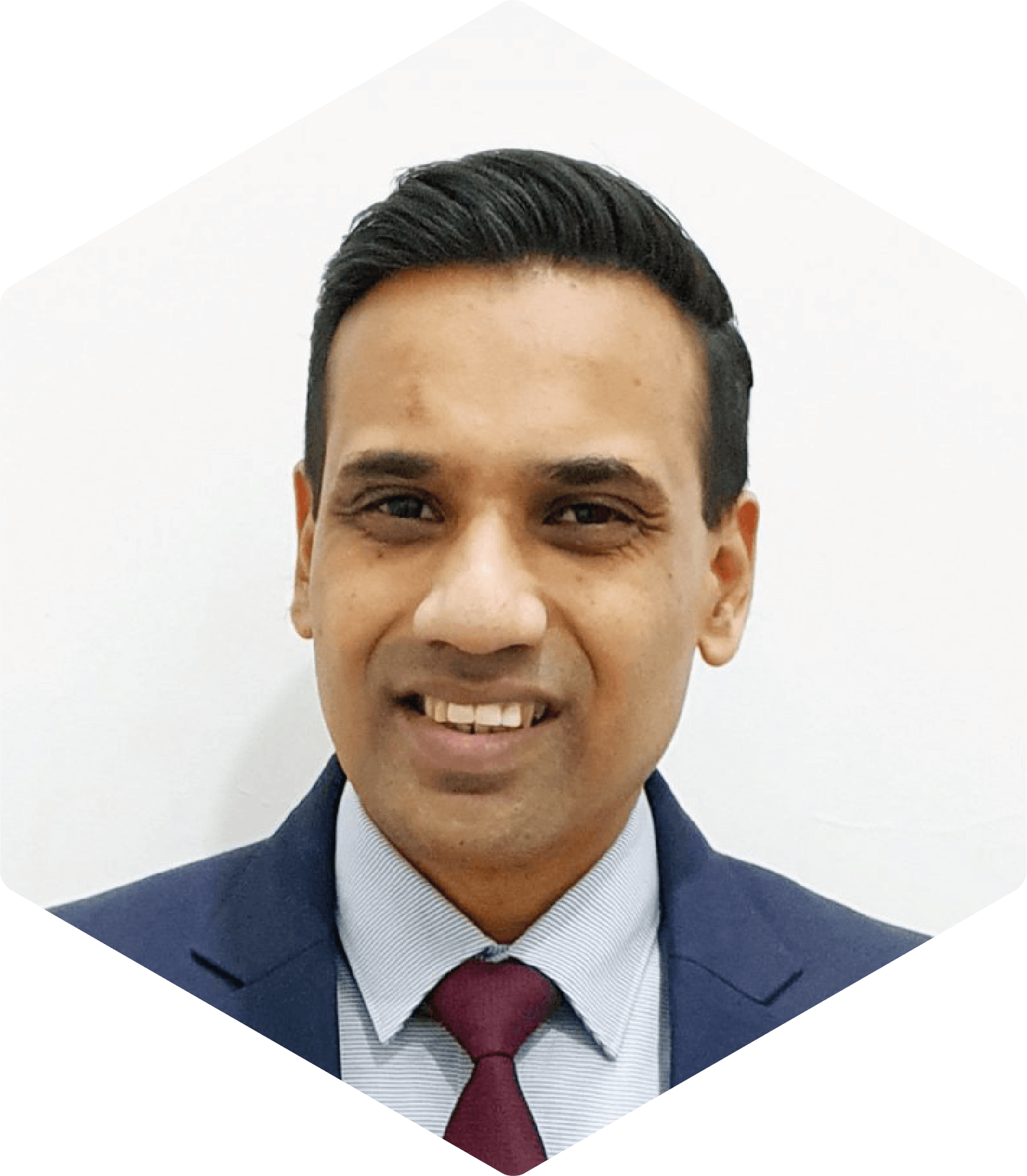
About Kunal:
Kunal Srivastava is the leader for Tech & AI in Deallus. Kunal is responsible for overall organisational Tech & AI strategy including implementing AI tools and platforms available in market as well as developing Deallus’ internal AI offerings.